With predictive analytics, big data becomes a big opportunity for B2B sales managers. This significant opportunity requires, however, a profound understanding of the sales situation, coupled with an understanding of big data mining models available.
A sales situation is best described by a list of key performance questions together with their performance indicators (KPI). Once you have listed the most pressing sales issues and have determined their corresponding KPIs, the data available and the data analysis methodology should follow.
In Business-to-Business, the most valuable data of Big Data is always at hand: sales transactions from an ERP System and sales activities from a CRM Software. The reason is that to make useful predictions, sales managers mainly need to find “positive” cases: for example, customers that have bought a product or accepted an offer. In other words, Predictive Analytics is about describing the relationships between past data and predictive outcomes.
Using ERP and CRM sales data available and Big Data Analytics methods, Predictive Analytics can, for instance, help sales leaders to spot hidden opportunities within existing B2B customers. Do you need some examples or ideas on where to being? Here we provide three data mining techniques for predictive sales analytics based on ERP and CRM sales data.
Example Number One: doing B2B marketing segmentation with a clustering method
Successful market segmentation is the key to aligning services and products of a company with the needs and demand of the market. As Andre Lingenfelser wrote in Vertriebsmanager: „ Big Data methods open up an enormous potential to make segmentation much more efficient for a sales manager.“
A clustering method groups customers together by their similarity, usually not driven by any particular purpose. Due to the “positive” nature of the past sales transactions (customers that bought a product or service), to successfully undertake a cluster analysis there is probably no better start than a company’s ERP sales data.
Sales managers can use cluster analysis to group existing buyers into different sets or “clusters”. Once sales managers arranged customers in groups, they can compare trends in each one and dig for more sales potential.
[bctt tweet=”Sales managers can compare customers clusters to discover cross-selling potential.”]
For example, Qymatix Predictive Sales Analytics Software runs an advanced arithmetical clustering function to cluster customers together. It then detects buyers in those clusters that have not fulfilled the same buying potential as their peers. It then ranks them, helping to prioritise sales activities.
Example Number Two: using an apriori algorithm to develop a cross-selling strategy
Since the most popular ERP systems (SAP, Oracle, MS Dynamics, among others) use transactional databases, Apriori algorithms can offer valuable buying insights with low effort.
Like the clustering example above, an Apriori algorithm can spot associations and learn rules among customers. Marketers know Apriori well for its useful application in market basket analysis in B2C (business-to-consumer).
For example, if several customers bought products A and B together, the algorithm will cluster them into a set or basket. Sales managers can then compare these assortments to spot new business opportunities and increase cross-selling predictively.
Moreover, sales leaders can use these sets or clusters to detect pricing inconsistencies amongst customers. For example, such an ERP data mining technique can find customers consistently paying prices below a given average and spot them in advance.
Use Case: Predictive Analytics reduced customer churn while increasing sales team satisfaction and engagement.
Example Number Three: implementing a customer behaviour model for sales forecasting
With Big Data analytics, the right sales action should go to the right customer at the right time. How to improve sales forecast based on customer behaviour? Sales managers can apply predictive analytics models using customer response and then undertake appropriate actions.
An ARIMA (autoregressive integrated moving average) algorithm influenced by CRM sales data is an excellent Big Data method for improving sales forecast based on customer behaviour. Improvements in sales forecasting can offer benefits not only for stock replenishment but also advantages in customer loyalty, reduced attrition and churn.
[bctt tweet=”An ARIMA algorithm mixed with CRM data can improve sales forecasting based on customer behaviour.”]
For example, running an ARIMA algorithm across ERP sales transactions while feeding it with CRM signals (i.e. calls, complaints, sales activities) sales leaders can spot hidden buying opportunities and significantly improve sales forecasting. Likewise, using such an advanced predictive sales analytics function, sales leaders can avoid customer attrition and churn and recognise their reasons earlier.
Use Case: How cross- and up-selling based on Qymatix Algorithms is helping a medical components manufacturer to sell more.
CALCULATE NOW THE ROI OF QYMATIX PREDICTIVE SALES SOFTWARE
B2B Predictive Analytics examples using Big Data – Conclusion:
Regardless of how big Big Data is, the definition of data mining and predictive analytics methods begins with the understanding of the kind of information the sales force needs to be successful.
Mining CRM and ERP data for Predictive Analytics is a process intended to explore past sales data in search of patterns and behaviours among different variables. Once sales managers have discovered those relationships, they can use them as a model to make accurate forecasts, identify new sales opportunities, and increase the efficiency of the sales force.
[bctt tweet=”In B2B sales situations, Big Data does not need to be big, it has to be useful.”]
There are many inspiring examples of Big Data analytics. It is, however, critical understanding that in Business-to-Business sales, Big Data does not need to be big.
CRM and ERP Data Mining is a good start for Predictive Analytics. ERP and CRM sales data is one of the most valuable datasets a company can analyse. Therefore, sales managers in B2B should first dig this sales data for useful insights. Clustering analysis, apriori and behaviour-based forecasting are three good examples of where to start generating value from data.
How relevant is Predictive Analytics a relevant Topic for your company? Comment below.
I want to know more about Qymatix Predictive Sales Software
Free eBook for download: How To Get Started With Predictive Sales Analytics – Methods, data and practical ideas
Predictive analytics is the technology that enables a look into the future. What data do you need? How do you get started with predictive analytics? What methods can you use?
Download the free eBook now.
- We will use this data only to contact you for discussing predictive sales KPIs. You can read here our declaration on data protection.
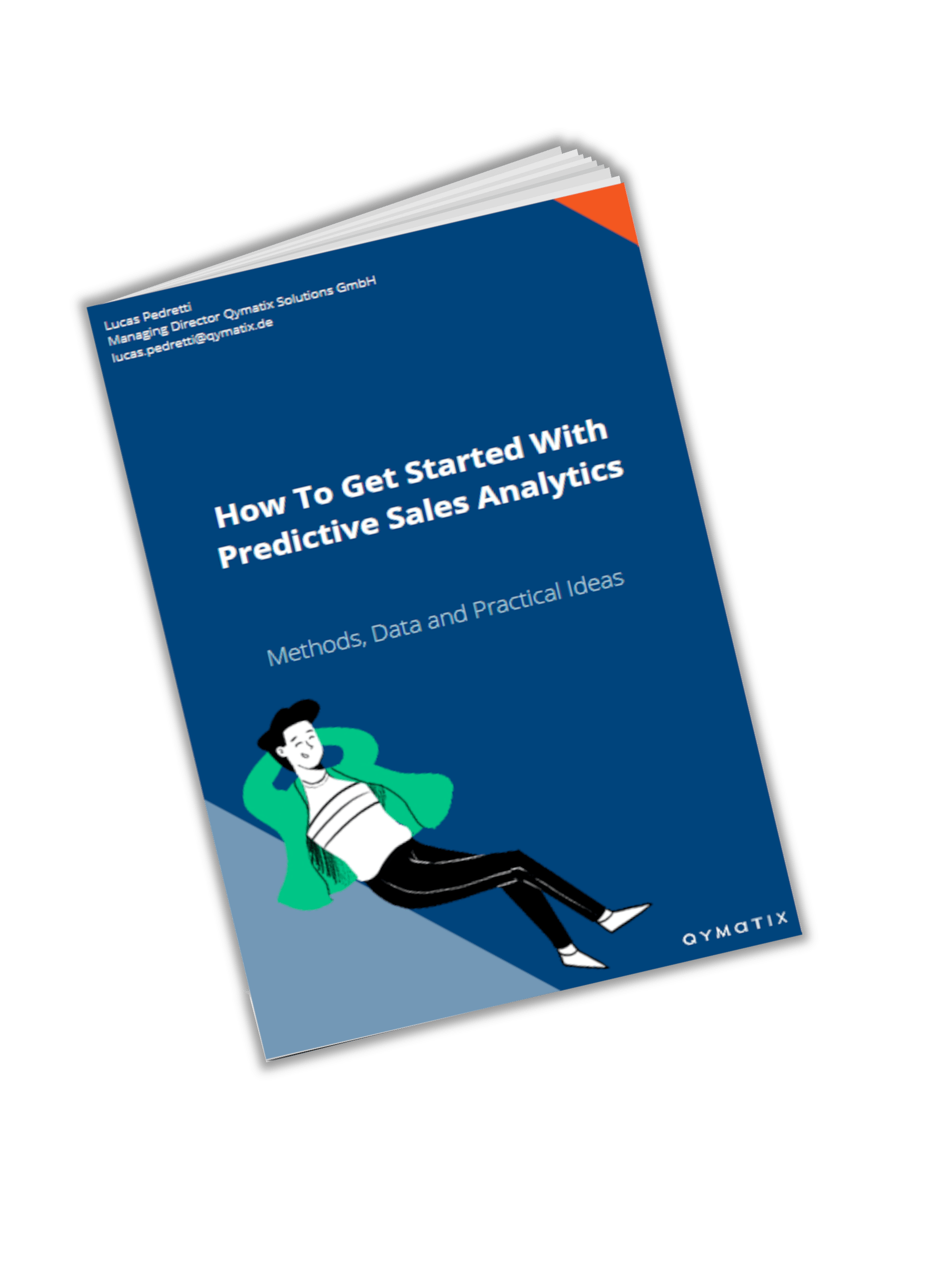
Also interesting:
The Top 5 ERP Sales Data Mining Techniques You Need in B2B Now
Further Read: